Curation
The first step for success begins here. Everyday, thousands of datasets are made public through open access publications. These are deposited in dedicated databases which permanently store them to be used by anyone.
However, data comes in different forms and flavors, and standard updates are constantly implemented when new the data is deposited, either increasing its discoverability or protecting sensitive information (eg GDPR).
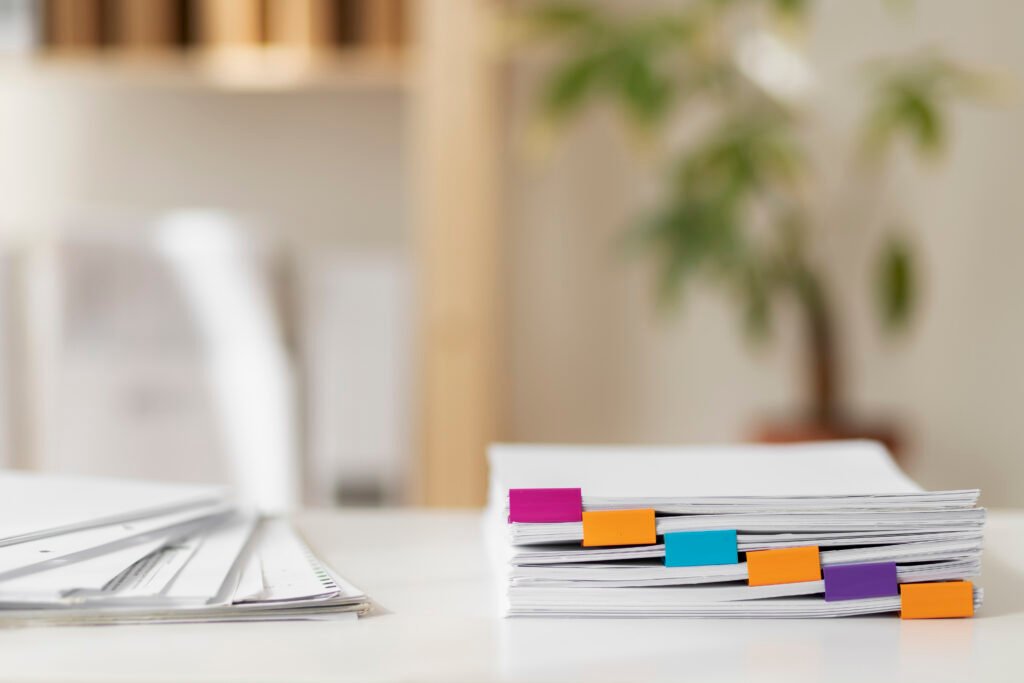
Metadata standardization
If you ever tried to utilize datasets produced from different labs, or even datasets from the same lab from different time points, you’ll quickly realize that each one is formatted differently. By collecting and curating thousands of samples, we identify the intrinsic patterns in the data and use it to push curation quality to perfection.
Our algorithms convert all free-text fields, including abbreviations and mispellings, to the correct ontology term. For example, we ensure that terms such as “Crons Disease”, “Crohn’s disease”, “Crohns ileitis”, “CD”, “IBD, small intestine” are all converted to the same term “Crohn disease” (MESH:D003424).
Filling the gaps
Doing bioinformatic analysis at the atlas-level allows us to identify and automatically assign new metadata not available at the original dataset. This can vary from assigning the biological sex to all samples, organs and cell type labels to all cells and samples.
A classical example is from a set of 28,000 samples (single-cell), about 2000 of them have the the biological sex assigned, that equals only 7% of all datasets! We can confidently assign the correct respective sex to all samples, saving you the trouble to do so and allowing you to include that information on your analysis!
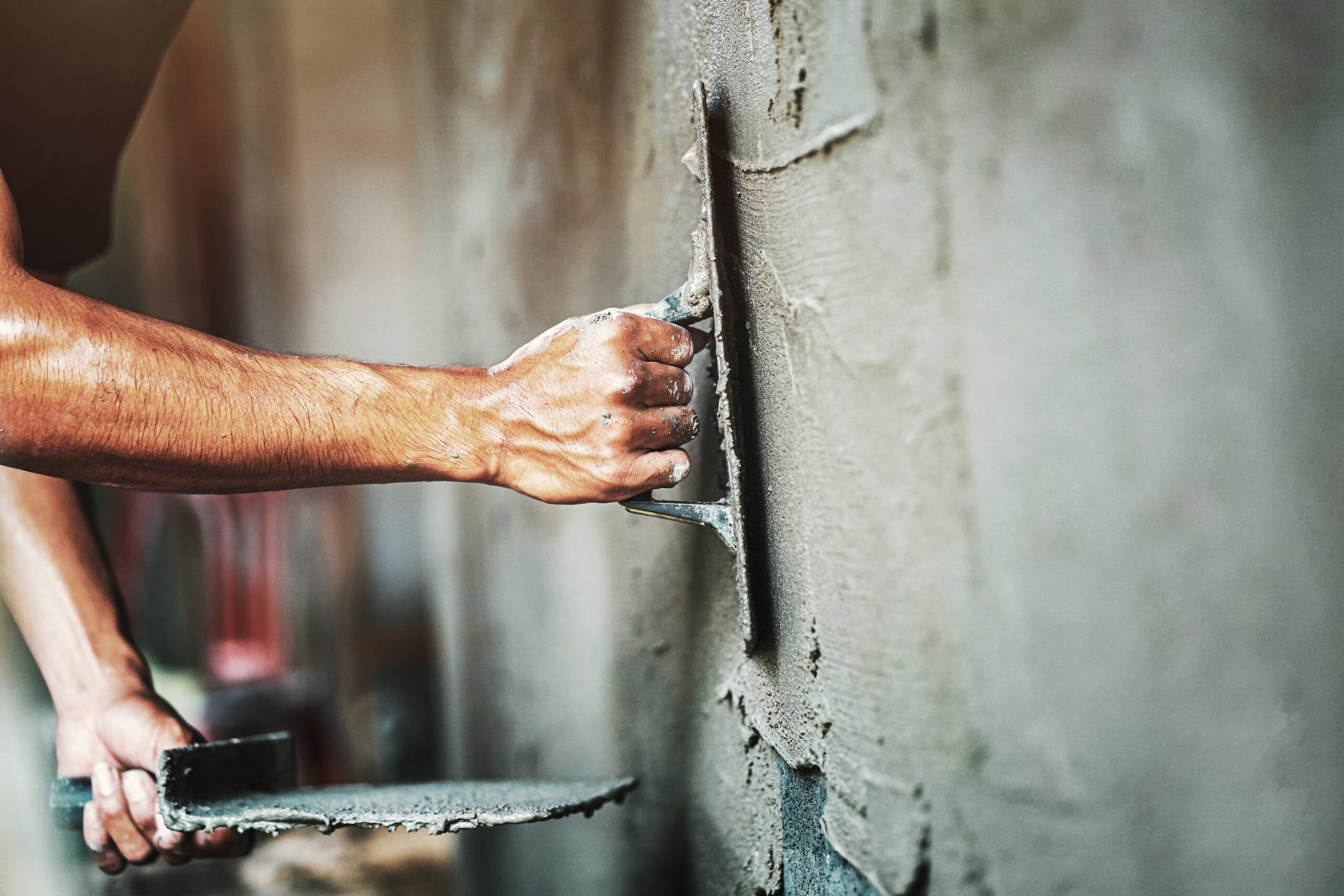
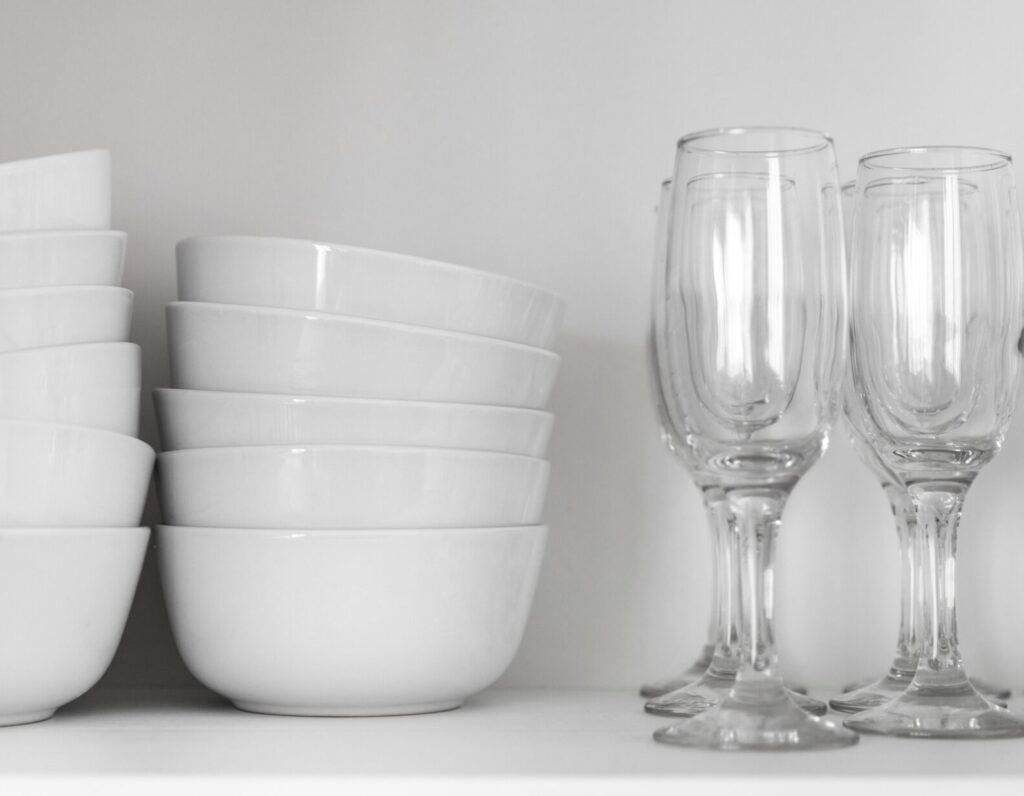
Data homogenization
Besides curation of metadata, we have developed algorithms that can scale linearly, which allows us to efficiently process any amount of datasets even with limited resources. That includes automatic ingestion of matrices saved in multiple formats, and conversion to current .h5ad standard.
Besides that, we also harmonize and map datasets annotated with different genomes. That means updating the gene names, symbols, ids, fixing errors in the original datasets and identification of incomplete or corrupted data.
For the community
During the process of conversion of all free-text entries to curated categories, we assign every name to an ontology term when possible. Just like in the example of multiple writing and synonyms exist, the naming across our whole database is consistent and has an identifier.
In reality, we are taking a step further and actually contributing to existing ontologies by adding new terms found by literature review and manual annotation of unlabeled cell types with known names. There are multiples examples of this, but even simple cases like “CD8 T cells” does not have an ontology while “CD4 T cells” does. Strange, no? We’ll do our best to help by filling those gaps in the ontology!
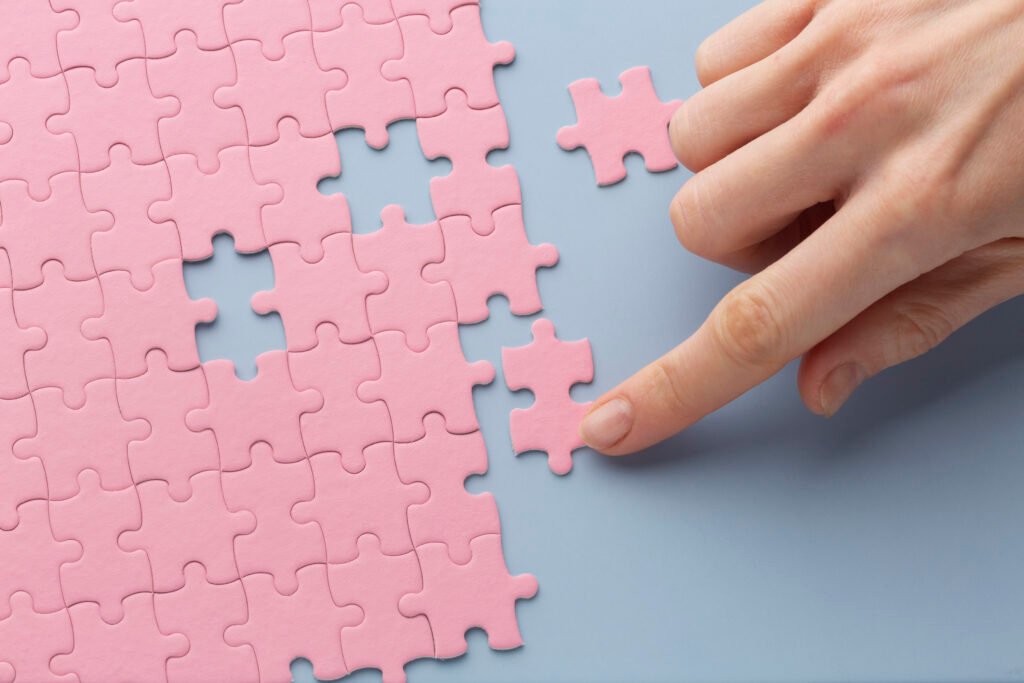
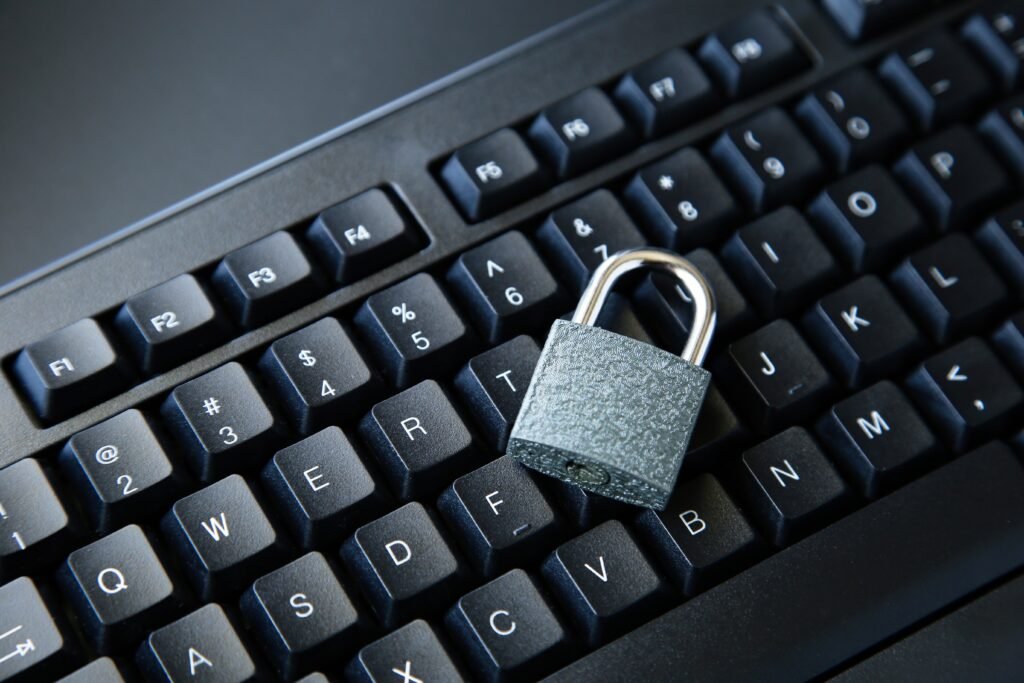
Sensitive data handling
We are committed to protecting sensitive information present in public repositories. On our path to making research data accessible and discoverable, we are always exposed to sensitive data, usually in the form of genetic sequences. This information will never be sold from us.
Instead, we extract only necessary information to allow a more detailed analysis and usage of the non-sensitive information in that precious sample, making it discoverable and accessible. All sensitive data is permanently deleted from our servers once extraction is done.